This talk explores the application of reinforcement learning (RL) in large-scale recommendation systems to optimize user retention at scale - the true north star of effective recommendation engines. We'll discuss how RL can learn patterns and attribute future retention behavior to content consumed in current sessions, providing a more holistic approach than traditional methods.
We'll share insights from implementing this strategy in a production environment serving millions of users, highlighting significant improvements in key retention metrics. The presentation will address major challenges, including noisy attributions and proving causality within the system.
Our findings demonstrate the potential of RL in creating more sustainable, user-centric recommendation systems across various digital platforms, with important implications for the future of personalized content delivery.
Key Takeaways:
- Using long-term rewards at different future horizons leads to incrementality in long-term metrics like Daily active users and sessions (will share results in the talk).
- Optimal to try different horizons and approaches since duration is a tradeoff between causality of the lever and correlation with the final long-term metric.
- High ROI of reward shaping to encode product intuition and strategy (e.g. private sharing)
- Lessons on model architecture, co-investment in infrastructure (GPU inference, user history processing, sequence modeling) required to derive benefit at scale.
Speaker
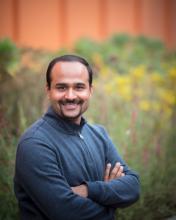
Saurabh Gupta
Senior Engineering Leader @Meta, Veteran in the Video Recommendations Domain, Helping Scale Video Consumption
Saurabh Gupta is a Senior Engineering Leader at Meta Inc. He leads the video recommendations core ranking organization which is directly responsible for serving personalized video recommendations to billions of users on Facebook. These video recommendations constitute one third of time consumed by all users on Facebook app. He is a veteran in the video recommendations domain and has helped scale video consumption by many folds on Facebook in the last 9+ years. His work focuses on building scalable retrieval systems, understanding user interests, building large scale ML models to predict user actions and delivering personalized and highly relevant video feeds involving short form and long form videos on parts of Facebook app. He received his M.S. in Computer Science with specialization in Machine Learning from Georgia Institute of Technology. He holds several US patents and many more in pipeline in areas of machine learning, recommendations and software engineering.
Speaker
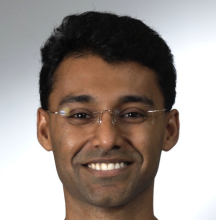
Gaurav Chakravorty
Uber TL @Meta, Previously Worked on Facebook Video Recommendations and Instagram Friending and Growth
Gaurav is an Uber TL at Meta Inc, previously in Facebook video recommendations and more recently in Instagram friending and growth. He has worked on end-to-end recommender system advances, including user retention modeling. Prior to this, he has led applied ML based initiatives at Discord and Google, and high frequency trading.