Discover the transformative impact of Generative AI and large language models (LLMs) on AI/ML advancements. We will delve into the latest trends and techniques for building modern ML systems and applications. The track will focus on three crucial themes: generative AI, trust, and the path to production.
Focus Areas:
- Generative AI for LLMs: Explore Retrieval Augmented Generation and its impact on LLMs.
- Trust and Efficiency: Mitigate risks and enhance the safety and efficiency of LLM-powered applications.
- Scalability and Optimization: Leverage modern compute stack for scaling AI/ML/LLM workloads.
- Data-Centric AI Applications: Master the path to production for data-centric AI applications.
Join us to explore these transformative areas that are shaping the future of ML/AI, and gain the knowledge to build more potent and dependable ML systems.
From this track
Chronon - Airbnb’s End-to-End Feature Platform
Tuesday Oct 3 / 10:35AM PDT
ML Models typically use upwards of 100 features to generate a single prediction. As a result, there is an explosion in the number of data pipelines and high request fanout during prediction.
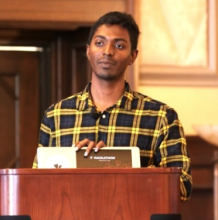
Nikhil Simha
Author of "Chronon Feature Platform", Previously Built Stream Processing Infra @Meta and NLP Systems @Amazon & @Walmartlabs
Defensible Moats: Unlocking Enterprise Value with Large Language Models
Tuesday Oct 3 / 11:45AM PDT
Building LLM-powered applications using APIs alone poses significant challenges for enterprises. These challenges include data fragmentation, the absence of a shared business vocabulary, privacy concerns regarding data, and diverse objectives among data and ML users.
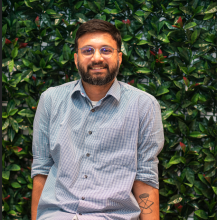
Nischal HP
Vice President of Data Science @Scoutbee, Decade of Experience Building Enterprise AI
Modern Compute Stack for Scaling Large AI/ML/LLM Workloads
Tuesday Oct 3 / 01:35PM PDT
Advanced machine learning (ML) models, particularly large language models (LLMs), require scaling beyond a single machine.
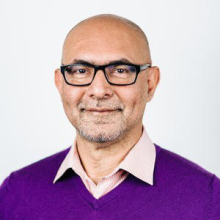
Jules Damji
Lead Developer Advocate @Anyscale, MLflow Contributor, and Co-Author of "Learning Spark"
Generative Search: Practical Advice for Retrieval Augmented Generation (RAG)
Tuesday Oct 3 / 02:45PM PDT
In this presentation, we will delve into the world of Retrieval Augmented Generation (RAG) and its significance for Large Language Models (LLMs) like OpenAI's GPT4. With the rapid evolution of data, LLMs face the challenge of staying up-to-date and contextually relevant.
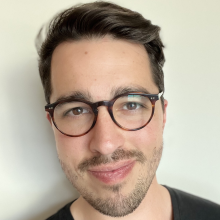
Sam Partee
Principal Engineer @Redis
Unconference: Modern ML
Tuesday Oct 3 / 03:55PM PDT
What is an unconference? An unconference is a participant-driven meeting. Attendees come together, bringing their challenges and relying on the experience and know-how of their peers for solutions.
Building Guardrails for Enterprise AI Applications W/ LLMs
Tuesday Oct 3 / 05:05PM PDT
Large Language Models (LLMs) such as ChatGPT have revolutionized AI applications, offering unprecedented potential for complex real-world scenarios. However, fully harnessing this potential comes with unique challenges such as model brittleness and the need for consistent, accurate outputs.
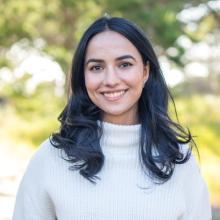
Shreya Rajpal
Founder @Guardrails AI, Experienced ML Practitioner with a Decade of Experience in ML Research, Applications and Infrastructure